Single sentence
Short version
- 앙상블은 성능 올리기 짱!
- 문제는 훈련할 때 너무 비싸고, deployment까지 고려하면 답없음 ;;;
- 누군가 앙상블을 압축하는 방식을 제안함
- 요거 가지고 조금 변형해서 해봤더니 괜찮음!!!!!
Middle version
Analogy
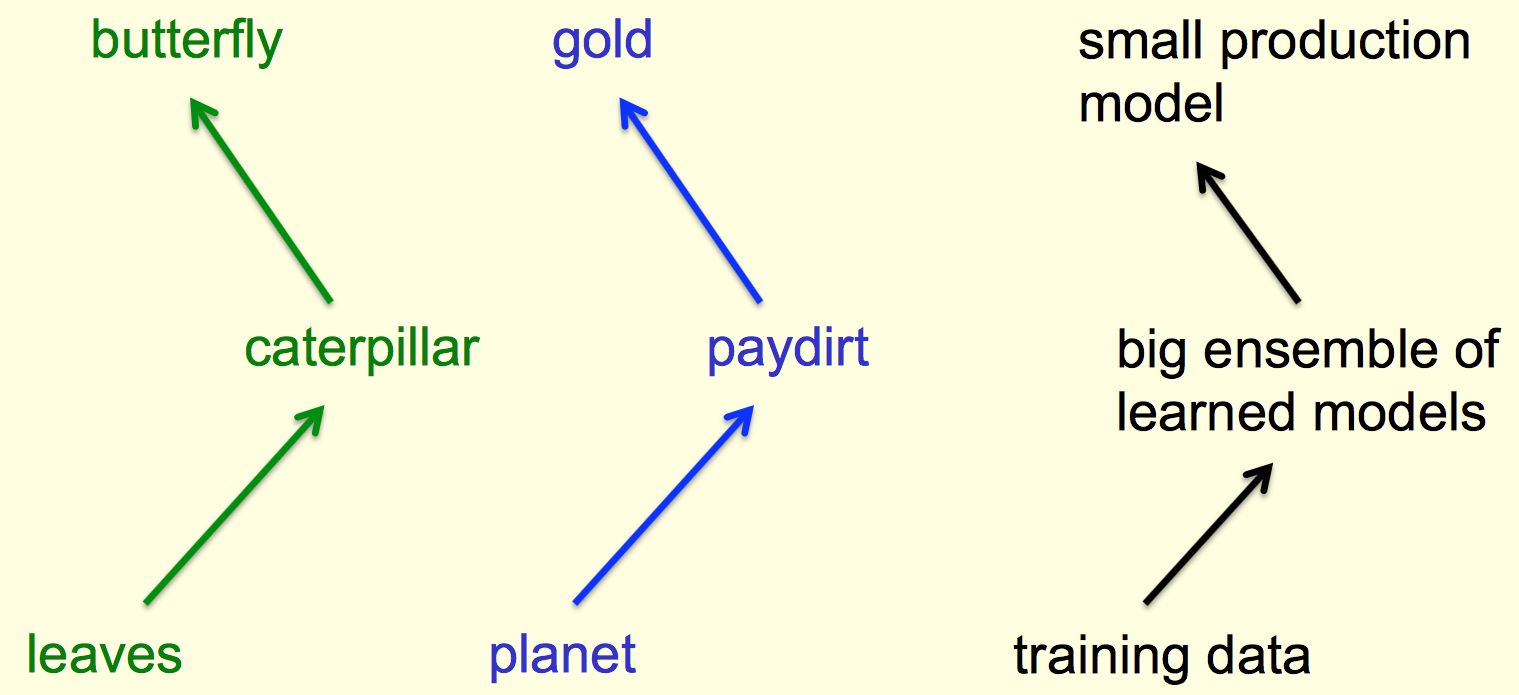
Key
앙상블을 하나의 함수로 보고, 이걸 학습하도록 해보자.
- Caruana et. al. 2006에 같은 아이디어지만 다른 방식으로 transfer learning 했었음.
Key: Softmax with Temperature
Hard targets: 기존의 ground truth (one-hot vector)
Soft targets:
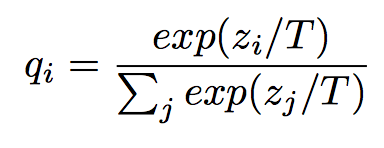
Concept
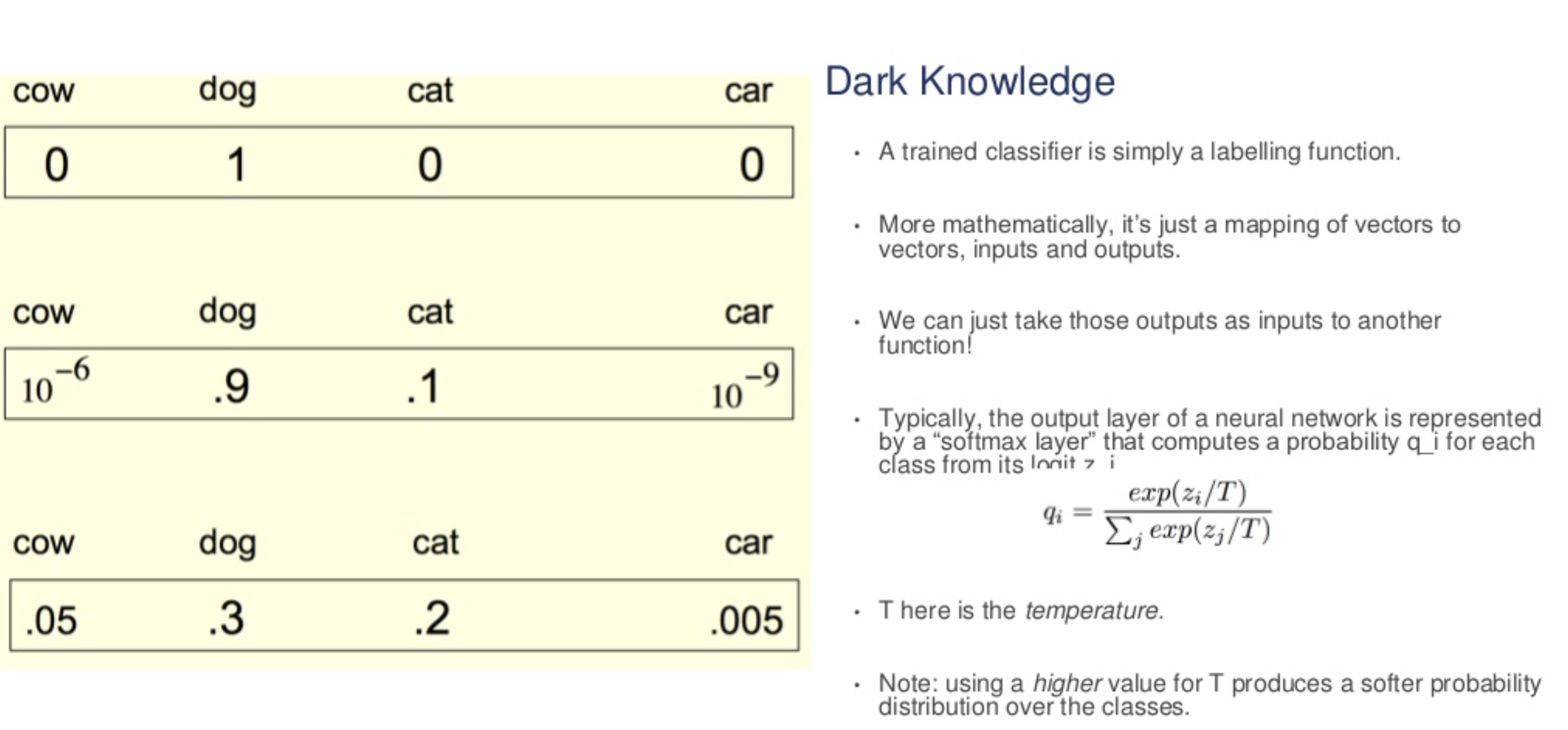
Distillation
기본적으로 NN은 softmax로 분류 기능을 하는데 세부적으로 보면 다음과 같다.
조금 다른 점은 \(T\)라고 하는 temperature가 추가되어 있다.
이게 RL이나 statistical mechanics 에서 사용하는 방식이라 함.
Distillation (2)
위키를 찾아보면

\(T\)를 기능적으로만 보면 값이 커지면 상대적으로 값들이 차이가 안날테니 even하게 빠질테고, 값이 작으면 (1보다 작으면) 조금의 차이가 더 강조되어 확률값으로 반영되게 된다.
값이 커질수록 나오는 확률 값들은 softer해진다고 볼 수 있다.
Distillation (3)
The same high temperature is used when training the distilled model, but after it has been trained it uses a temperature of 1.
이 내용을 보면 압축 모델 (distilled model)을 훈련할 때는 high temperature로 하고 사용할때 deploy할 때는 1로 설정하고 사용하는 걸로..
앙상블 모델 가지고 transfer set이라는 것을 만든다. 그런데 이걸 만들 때 쓰는 것 자체가 high \(t\)를 가지고 만든다. 그리고 그걸 가지고 distilled model을 훈련한다.
In the paper:
hidden: 300 > -> 8 이상의 \(t\)에서 괜찮음.
hidden: 30 -> 2.5 ~ 4 정도의 \(t\)가 괜찮음.
Efficient Ensemble
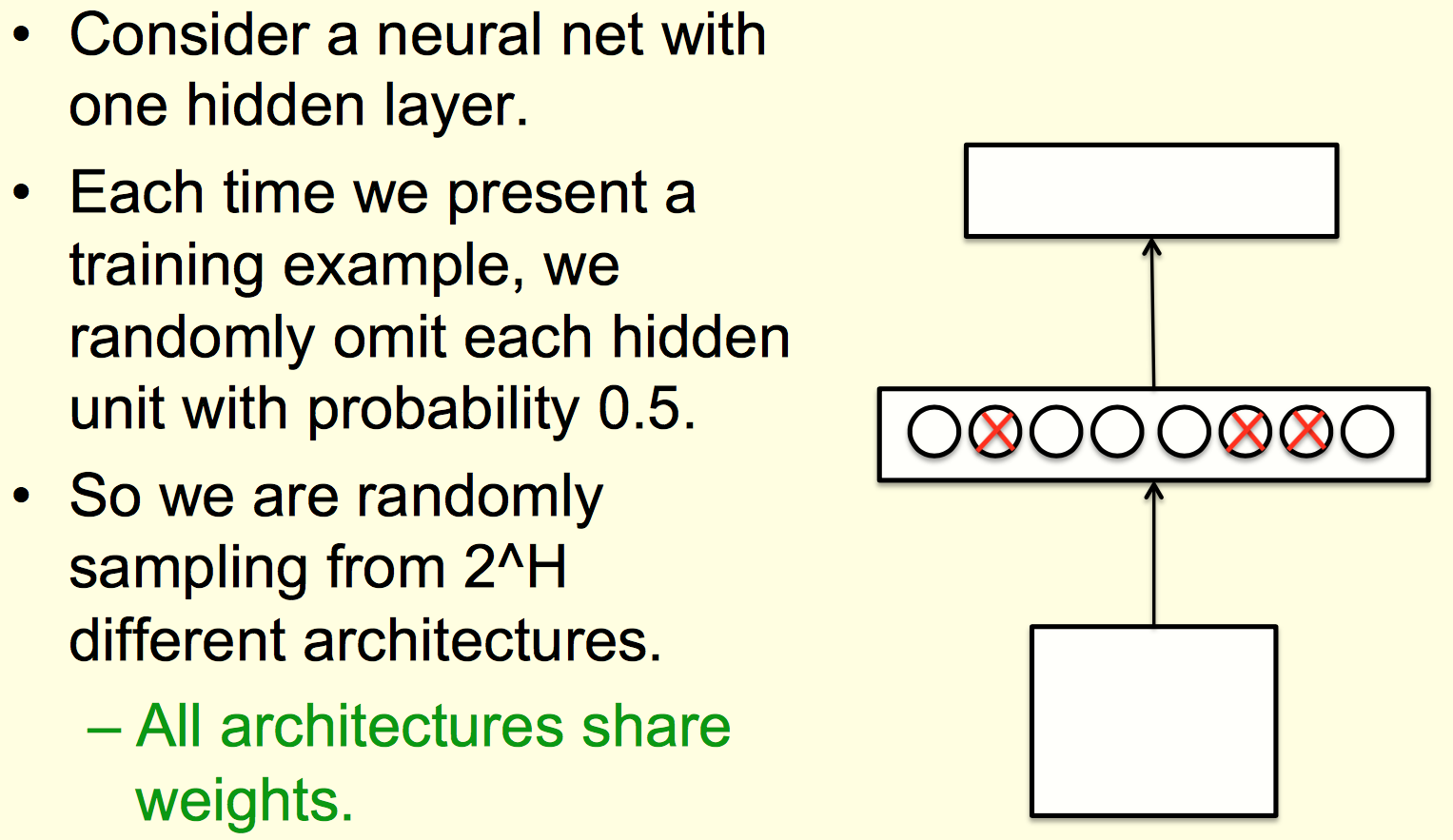
Efficient Ensemble (2)
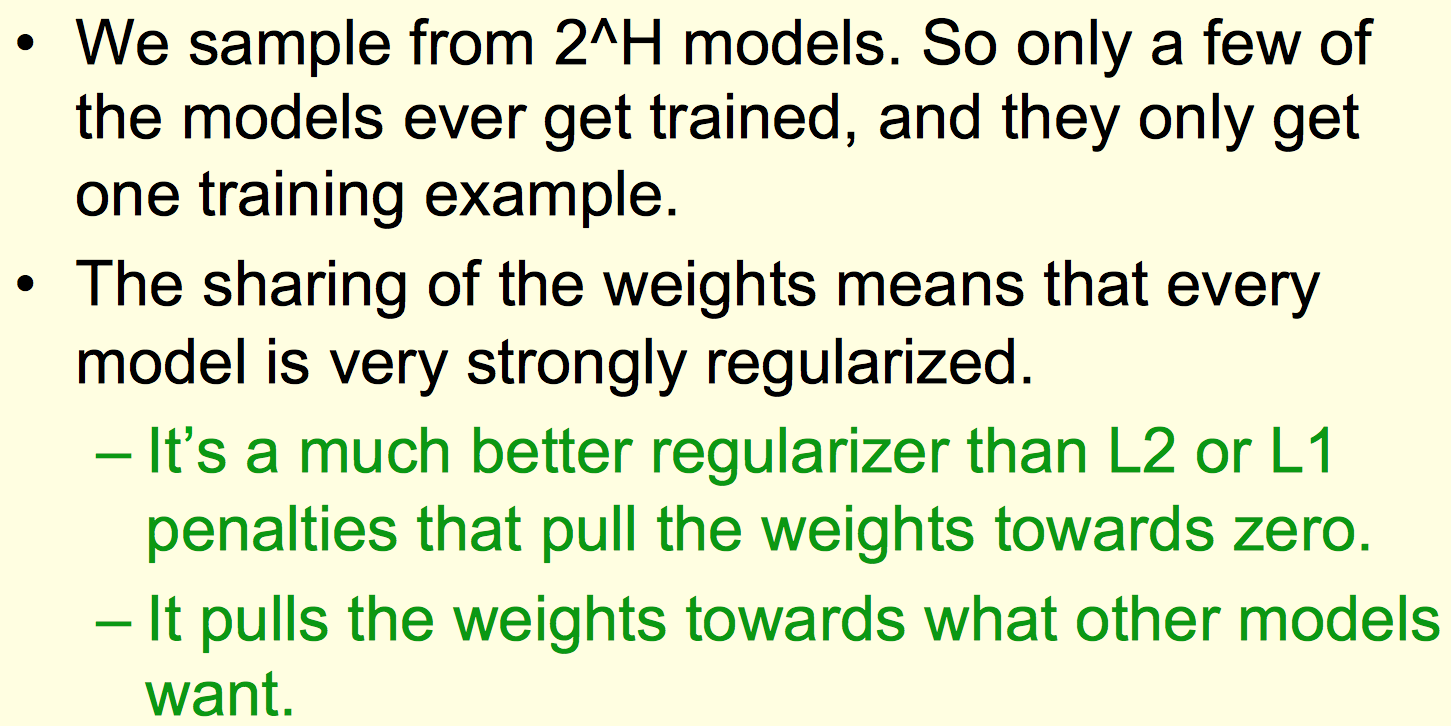
Efficient Ensemble (3)
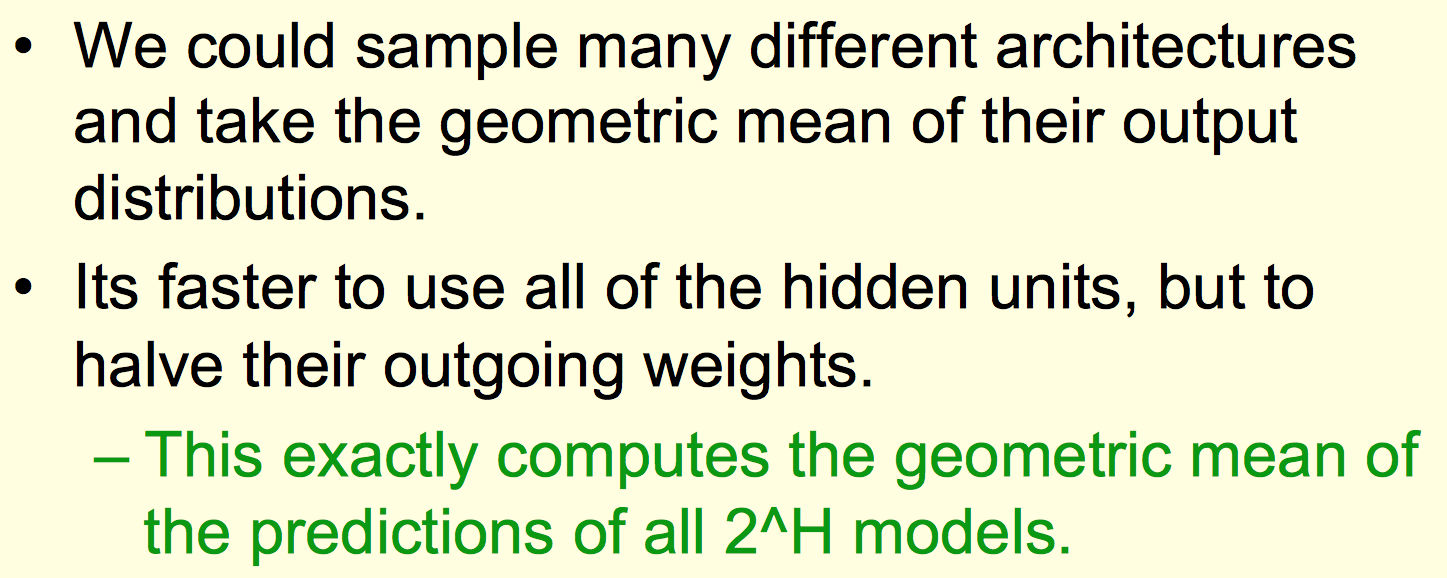
Efficient Ensemble (4)
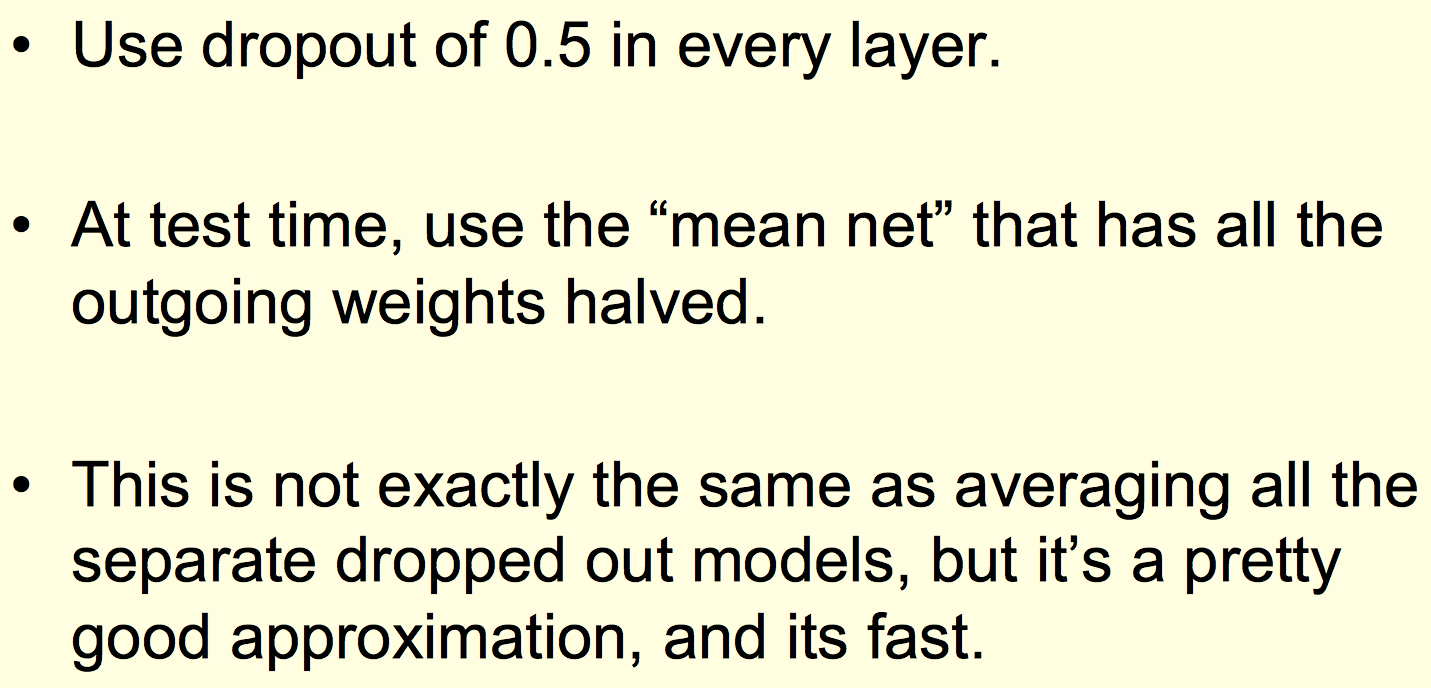
실험 1
Small model on same dataset
- MNIST
- Model: 784 -> 800 -> 800 -> 10 (with ReLU)
- Dropout Model: 784 -> 1200 -> 1200 -> 10
- Distilled Model: 784 -> 800 -> 800 -> 10 (T: 20)
Small model on small(sub) dataset
- MNIST
- Model: 784 -> 800 -> 800 -> 10
- Transfer Model: same architecture, No 3 data
- Transfer Model: same architecture, Only 7, 8 data
- 87% correction over all classes (0-9)
Adaptation
- Google production speech model
- get 6/7 accuracy on only 3% of dataset
실험 2
음성인식에 적용.
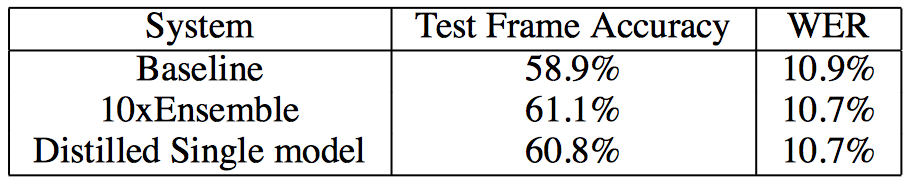
결과를 보면 하나의 모델로 만들었음에도 앙상블처럼 성능향상이 있다.
실험 3
일부 데이터

결과를 보면 3프로 가지고 했음에도 많이 성능을 따라감을 확인할 수 있다.
Conclusion
- Ensemble이나 large 에서부터 작은 모델로 학습 잘 된다.
- 이를 위해 soft targets을 훈련에 사용할 것을 제안했다.
- 나머지(specialist)는 잘 모르겠음;;;;
References
deep model compression
dark knowledge